Forthcoming and Online First Articles
International Journal of Information and Communication Technology
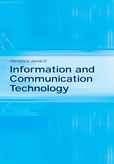
Forthcoming articles have been peer-reviewed and accepted for publication but are pending final changes, are not yet published and may not appear here in their final order of publication until they are assigned to issues. Therefore, the content conforms to our standards but the presentation (e.g. typesetting and proof-reading) is not necessarily up to the Inderscience standard. Additionally, titles, authors, abstracts and keywords may change before publication. Articles will not be published until the final proofs are validated by their authors.
Forthcoming articles must be purchased for the purposes of research, teaching and private study only. These articles can be cited using the expression "in press". For example: Smith, J. (in press). Article Title. Journal Title.
Articles marked with this shopping trolley icon are available for purchase - click on the icon to send an email request to purchase.
Online First articles are published online here, before they appear in a journal issue. Online First articles are fully citeable, complete with a DOI. They can be cited, read, and downloaded. Online First articles are published as Open Access (OA) articles to make the latest research available as early as possible.
Register for our alerting service, which notifies you by email when new issues are published online.
International Journal of Information and Communication Technology (22 papers in press) Regular Issues
Abstract: With the popularisation and development of ice and snow sports, the measurement and evaluation of athletes sports parameters are of great significance in improving their competitive level and avoiding sports injuries. This article proposes a linear motion parameter measurement and evaluation method for ice and snow athletes based on acceleration sensors. This method collects real-time acceleration data during exercise by wearing acceleration sensors, and uses data fusion and filtering techniques to extract linear acceleration and velocity changes of athletes. Furthermore, the Kalman filtering algorithm is used to optimise the noisy data in order to improve its accuracy and stability. And use support vector machine (SVM) algorithm to classify and evaluate the athletes motion state. The experimental results show that this method can accurately measure the linear motion parameters of ice and snow athletes, efficiently evaluate their sports status. Keywords: acceleration sensor; linear motion parameters; exercise assessment; Kalman filter; support vector machine; SVM.
Abstract: In this work, a framework based on the combination of generative adversarial network (GAN) and Drools rule engine, Drools-GAN, is suggested to increase the quality of reproduction and generating of traditional festival celebration elements in animation. Combining a generative adversarial network with a rule engine guarantees that the produced images not only meet particular festival aspects and rule criteria but also of great quality. We confirm the advantages of the Drools-GAN framework in terms of image quality, rule compliance, and synergy of its modules by means of a sequence of studies comprising comparison and ablation experiments. The testing results reveal that the framework can guarantee that the produced results fit the preset themes of holiday celebrations and efficiently increase the quality of image generating. The framework shows the possibility for application in animation production and offers a fresh solution for the mix of rule-based image generating. Keywords: generative adversarial network; GAN; Drools rule engine; festive elements; image generation; animation creation.
Abstract: As the big data technique rapidly develops, the demand for inter-agency cross-domain data sharing is growing, but there is a risk of unauthorised access in cross-domain data sharing. To this end, this paper first improves the ciphertext policy attribute-based encryption (MCACP-ABE), which achieves fine-grained protection of cross-domain data by authorising cross-domain third parties and attribute authority centres, and introduces the accountability tracking module. On this basis, fine-grained data cross-domain access control (AC) policies are designed. The policy designs a cross-domain AC structure based on MCACP-ABE, which realises fine-grained data access protection through a cross-domain negotiation component, a rule mapping component, and a cross-domain encryption component. The security analysis and simulation outcome imply that the offered policy not only satisfies indistinguishable security under chosen ciphertext attack (IND-CCA) but also has high cross-domain communication efficiency, which improves the security and usability of data cross-domain access. Keywords: cross-domain access control; ciphertext policy attribute-based encryption; accountability tracking; cross-domain negotiation; rule mapping.
Abstract: With the booming development of digital economy, the Internet has become an important platform for social emotions to converge. The detection of online public opinion is of vital importance to social stability. Focusing on the issue that current research cannot dynamically extract sentiment features, firstly, we use the dual-channel deep learning model to obtain the global and local sentiment features of the network comments respectively, enhance the sentiment features by the improved attention mechanism (EAM), and fuse the features to classify the sentiment of the public opinion using softmax. Subsequently, this paper classified the network opinion into levels, and through the results of sentiment classification and combined with many indicators, a comprehensive calculation was carried out to obtain the public opinion detection level. Experimental outcome on two Twitter datasets show that the proposed method improves the weighted average F1 by 4.12%-19.6%. Keywords: web public opinion detection; deep learning; attention mechanism; text sentiment categorisation; opinion rating calculation.
Abstract: Traditional image style transfer methods cannot preserve the content and structural features of the original image while maintaining a specific style. To preserve the semantic information of the original image, a multi-scale cycle-consistency generative adversarial network model is developed. This model can enable innovative style transformations while maintaining the original artistic characteristics of illustrations. This model can better capture and integrate detailed features of different styles by performing style transfer at different resolution levels. The results showed that the proposed model improved the inception score by 1.755 and 0.122 respectively compared to the other two methods, indicating a significant improvement in image generation quality and superiority in image generation. When the low-level texture feature loss, adversarial loss, and high-level concept feature loss were removed, the Frechet inception distance value significantly increased from 73.72 to 102.28, an increase of approximately 38.74%, emphasising the role of these components in the model. The model proposed in this study achieves diverse style transfer and can maintain high image quality when generating stylised images, providing artists and designers with greater creative inspiration and choice space. Keywords: multi-scale CycleGAN; generative adversarial networks; illustration images; style transfer; classification.
Abstract: Robot route planning becomes crucial in intelligent navigation systems given the fast advancement of automation technologies. Concerning efficiency and resilience in handling challenging dynamic settings, traditional path-planning approaches have several restrictions. Based on variational autoencoder (VAE) and generative adversarial network (GAN), this work presents a path planning model, VAE-GAN PathNet, to handle this challenge. By combining the benefits of VAE in latent space modelling and the capacity of GAN in path optimisation, the model essentially increases the quality, smoothness and obstacle avoidance performance of path planning. This work uses the Stanford Drone Dataset and the ROS Path Planning Dataset to validate the efficacy of the model using trials. In terms of path length, obstacle avoidance performance, path smoothness and computing time, VAE-GAN PathNet beats conventional path planning algorithms experimental data demonstrate. Keywords: variational autoencoder; VAE; generative adversarial network; GAN; path planning; obstacle avoidance; path smoothness.
Abstract: With an aim to increase learners learning efficiency and experience, this paper suggests a personalised foreign language learning path suggestion technique based on subject knowledge graph. Using a knowledge graph in the domain of foreign language studies, integrating multidimensional knowledge points such as vocabulary, grammar, and pragmatics, and revealing their correlations and hierarchical structures, a fusion recommendation algorithm based on multiple learning factors was designed. The method improves learning path recommendation by thoroughly considering the sequence and similarity of knowledge items. According to the trial data, this approach can help students master information points rather successfully, optimise the length of learning paths, and significantly enhance learning interest and enthusiasm, providing important references for the design of foreign language learning systems. Keywords: knowledge graph; recommended learning paths; similarity of knowledge points; cognitive diagnosis; collaborative filtering.
Abstract: To tackle the challenges of recognition difficulties and constrained computational resources in Pu'er tea intelligent harvesting, this research develops an optimized, resource-efficient object detection algorithm built upon the YOLOv8n architecture for detecting tender shoots of Pu'er tea. The methodology incorporates three primary enhancements: First, replacing the standard Conv module with the Adown down-sampling component enhances detection precision, significantly boosts processing speed, and minimizes model complexity. Second, modifying the detection head to the LADH configuration cuts down parameter volume, further streamlining the model. Third, integrating the AFGC attention mechanism refines detection accuracy. Experimental outcomes reveal that the optimized model achieves a 0.7% increase in mean Average Precision (mAP), accelerates detection speed by 482.9 FPS, and reduces model size by 1.9 MB compared to the baseline YOLOv8n. This work provides a technical foundation for advancing intelligent harvesting systems tailored for Pu'er tea cultivation. Keywords: the intelligent harvesting of Pu'er tea; target detection; YOLOv8; lightweight.
Abstract: This study explores the integration of fuzzy logic in an adaptive assessment system for English language teaching (ELT), aimed at providing personalised and dynamic evaluations of students language proficiency. The system evaluates various linguistic components such as grammar, vocabulary, fluency, and pronunciation, by converting these inputs into fuzzy sets and applying a fuzzy inference system to assess overall proficiency. The study evaluates the system through pilot testing, accuracy comparison with traditional evaluation methods, and usability testing. The results demonstrate a high correlation (r = 0.92) between the fuzzy logic-based scores and traditional human evaluations, as well as strong test-retest reliability (r = 0.93), supporting the systems validity and consistency. This study contributes to the field of adaptive language assessment by demonstrating the potential of fuzzy logic in providing personalised, reliable, and efficient evaluation systems in ELT. Keywords: fuzzy logic; adaptive assessment; English language teaching; ELT; personalised learning; language proficiency evaluation.
Abstract: The amount of user-generated data is growing with the fast expansion of social networks and e-commerce platforms; so, how to recognise and evaluate user values from these enormous amounts becomes a crucial study issue. In this study, a hybrid technique framework based on the combination of LDA topic model and DBSCAN clustering algorithm is provided for effective analysis of user values. First, the LDA model mines the possible themes of user-generated text data; then, the DBSCAN clustering method is applied to classify the behavioural traits of several user groups. Strong scalability and universality as well as accurate identification and classification of user values are shown by experimental validation on two real datasets of the proposed method. Better results in multi-dimensional user values analysis are obtained by the hybrid technique based on topic model and cluster analysis than by the conventional single model approach. Keywords: social networks; user values; topic modelling; latent dirichlet allocation; LDA; DBSCAN; cluster analysis.
Abstract: Course evaluation has evolved into a significant metric of educational quality given the explosive growth of online learning. Traditional sentiment analysis techniques still have some difficulties handling multidimensional sentiment expressions in online course evaluations even if they have some success with social media and review data. In order to increase the accuracy of sentiment analysis in online course evaluation texts, we present in this work a textual sentiment analysis approach based on the attention mechanism of emoticons. First, this work integrates text information with emoji as a necessary input feature for sentiment analysis to provide multimodal sentiment classification. Especially when dealing with online course evaluation texts with several sentiment dimensions, which has great benefits and provides more accurate course evaluation data for online education platforms, the model suggested performs well on several benchmark datasets and outperforms conventional sentiment analysis methods by means of experimental validation. Keywords: emoticons; attention mechanisms; text sentiment analysis; online course evaluation; sentiment classification.
Abstract: Currently, residential area renovation in urban planning is facing many problems. Traditional sensitivity analysis methods are often difficult to handle. Therefore, this article proposes a comprehensive method that combines Random Forest and Transformer models. Firstly, the random forest algorithm is used to evaluate the feature importance of multidimensional influencing factors in residential area renovation and identify key influencing factors; Subsequently, leveraging the advantages of the Transformer model, the complex nonlinear relationships and spatiotemporal dependencies between key factors were processed, improving the accuracy and interpretability of sensitivity analysis. Through empirical analysis, the effectiveness and superiority of this method in sensitivity analysis of residential area renovation have been verified, providing more scientific and accurate decision support for urban planning. Research has shown that the combination of random forest and Transformer can provide a new approach for sensitivity analysis in complex planning tasks. Keywords: random forest; transformer; city planning; sensitivity analysis.
Abstract: This study presents a reinforcement learning (RL) framework for real-time decision-making and resource optimisation in education. It integrates various data streams, such as learner performance, engagement, and institutional constraints, to enable precise and scalable decisions in diverse educational environments. The framework is assessed using three metrics: optimisation efficiency (OE: +32.1%), real-time decision accuracy (RTDA: +-28.4%), and equity distribution index (EDI: +26.7%). A comparison with heuristic-based models shows a cumulative improvement of +29.1% across all metrics. Powered by a deep neural network and optimised using policygradient techniques, the framework focuses on scalability and fairness in resource allocation. Validation with real-world datasets demonstrates its adaptability and robustness. This research lays a solid foundation for AI integration in educational systems, offering a new benchmark for transforming resource allocation and decision-making processes in academia. Keywords: data analytics; machine learning; reinforcement learning; decision making; resource optimisation; academic performance.
Abstract: A multi-UAV flight-path planning method is developed to provide communication services to user ships in blind maritime communication zones. The developed approach considers several limitations, such as the maximum flight speed and flight range. Owing to the limited energy and transmission range of UAVs, communication resources may be distributed unevenly, which could result in communication inequality. To address these problems, an optimisation problem is created to maximise the combined metrics of communication fairness and UAV energy efficiency. Considering the complexity of solving this optimisation problem, a deep-learning algorithm, DRL-1, is proposed. DRL-1 optimises the multi-UAV flight path-planning problem by utilising Ape-X and RNN based on the MADDPG method. Simulation result 1 demonstrates that the proposed optimisation algorithm effectively enhances the UAVs energy efficiency and communication fairness. Simulation result 2 shows a significant improvement in UAVs energy efficiency and communication fairness as the number of UAVs increases. Keywords: maritime communication; unmanned aerial vehicle; UAV; trajectory planning; Markov decision process; MDP; deep learning; multi-agent deep deterministic policy gradient; DDPG.
Abstract: Teacher effectiveness needs to be examined to improve the quality of education. However, traditional evaluation methods are found to have subjectivity and difficulty in the scalability and integration of data. Recent advancements in artificial intelligence (AI) and natural language processing (NLP) offer potential solutions. Building on the discussion of traditional quantitative and qualitative methods of English teacher evaluation, this study proposes a transformer-based framework for integrating qualitative feedback and quantitative metrics to optimise English teacher evaluations. An objectivity tool model that combines BERT for NLP processing and Shapley additive explanations (SHAP) for transparency, making objectivity easier. The approach was validated as a pilot study involving 100 English teachers at ten schools. Qualitative feedback contributed 30%, and RMSE (0.50) and R2 (0.95) were the lowest values for the transformer-based model. Stakeholders highly reported accuracy and interpretability as being good. The proposed framework offers a scalable and explainable solution to the classical approach's limitations. It shows how AI-driven evaluation systems can enhance teaching quality and assist in data-driven educational decisions. Keywords: teacher evaluation; transformer-based framework; natural language processing; NLP; explainable AI; XAI; qualitative feedback analysis; teaching effectiveness; scalable evaluation systems.
Abstract: With the rapid development of the internet, electricity meter engineering operation has become one of the most popular information dissemination electricity meter engineering operations nowadays, and the electricity meter engineering operation data on the internet is constantly increasing, and the following problem is that the improper use of electricity meter engineering operation is also becoming more and more extensive. The motion compensation module uses adaptive separable convolution to replace the traditional optical flow estimation algorithm, which can well handle the curve motion problem between pixels that cannot be solved by the optical flow method. For different electricity meter engineering operation frames, the motion compensation module predicts the convolution kernel that conforms to the image structure and the local displacement of the pixel, and realises the motion offset estimation and pixel compensation of the pixels of the following frame by means of local convolution. It is trained and tested on the same training set and test set as the current state-of-the-art multi-frame quality enhancement (MFQE) algorithm. The experimental results verify the proposed network has a good effect of electricity meter engineering operation artefacts. Keywords: electricity meter engineering operation compressed; deep learning; convolution; multi-frame quality enhancement; MFQE.
Abstract: The emergence of eSIM card is another evolution of SIM card, and it is also in line with the technical development direction and market demand of SIM card from large to small and from real to virtual. This paper aims to combine 5G communication technology to study the over-the-air programming and real-name authentication technology of eSIM, and proposes a new random access design for the long delay and rapid timing advance (TA) change characteristics of low-orbit satellite communication system. Moreover, this paper proposes a random access preamble structure based on polarity expansion and a new TA adjustment strategy based on downlink tracking. In addition, this paper designs a random access response data structure suitable for LEO satellite environment at the protocol layer, and builds a blockchain eSIM solution architecture design system based on 5G communication. Finally, this paper verifies the effectiveness of the technology proposed in this paper combined with experimental tests, thus providing a theoretical reference for subsequent related research on the popularisation of eSIM to user groups. Keywords: 5G; communication technology; eSIM; over-the-air programming; real-name authentication.
Abstract: The goal of style transfer is to apply the artistic features of a style image to a content image while maintaining the content image's structure. Traditional methods often use CNNs and residual blocks, but their limited receptive field struggles to capture long-range feature dependencies, leading to repetitive local patterns. Residual blocks can also cause interference between style and content representations. To address these issues, we introduce a pseudo-coordinates graph convolutional generative adversarial network (PGC-GAN), which consists of two branches: one for extracting style and another for style transfer. The style extraction branch represents style features as a graph and uses graph pooling to remove redundant information. The style transfer branch encodes these features into pseudo-coordinates, enabling flexible relationships between pixel nodes and long-range feature aggregation without disrupting the content image's structure. Experimental results demonstrate that PGC-GAN significantly improves artistic style transfer compared to existing methods. Keywords: art style transfer; graph convolution; pseudo-coordinates; GAN.
Abstract: Current research on charging station planning overlooks the evolution of power distribution networks and oversimplifies charging demand without considering the traffic characteristics of electric vehicles (EVs), leading to voltage deviations due to high charging loads. This paper proposes a bi-level optimisation model to simulate EV charging demand based on road networks. Charging demand is forecasted through road network simulation, and simplified charging needs are clustered with road weights. The model then optimises the power distribution network topology, as well as the location and capacity of charging stations. The upper level optimises the power network structure, while the lower level optimises the layout and capacity of charging stations. Case studies show that the clustering algorithm based on road weights effectively simplifies the data while retaining the spatiotemporal characteristics of charging demand. The proposed bi-level planning model significantly mitigates voltage deviations caused by high charging loads. Keywords: charging station planning; distribution network planning; clustering; electric vehicles; EVs; bilevel optimisation; distribution network; article swarm optimisation.
Abstract: In autonomous driving and computer vision, 3D object detection plays a critical role but faces challenges related to the effective extraction and integration of multi-view features. The existing BEVFormer model, which uses CNNs to convert images into a bird's-eye view (BEV), shows potential but struggles to capture fine-grained details and multi-scale information, especially in high-resolution, complex scenes. To address these limitations, we propose the MultiCAN-DEBEV model, which integrates the MSF-DySample, GCAF, and MSDE modules. These modules improve the handling of multi-scale features, enhance feature expressiveness, and strengthen detail representation. Experiments on the nuScenes dataset show significant performance improvements, and the modular design ensures broad adaptability to other 3D detection models. Keywords: computer vision; 3D object detection; BEV object detection; autonomous driving.
Abstract: Emotional evaluations can serve as indicators to guide the improvement of online travel service quality. This paper proposes a model based on a dual-stream graph attention fusion network. To prevent the introduction of noise unrelated to the subject matter, the network encodes the aspect texts and integrates them into the image-text embeddings through cross-attention mechanisms. Furthermore, the network incorporates a dual-stream interactive masking graph neural network to effectively align and fuse the deep features of multimodal image and text data. This allows the image data and textual data to provide rich supplementary information for each other, thereby enhancing the model's accuracy in sentiment analysis. In simulation comparisons with various state-of-the-art methods on real-world multimodal travel datasets, the results demonstrate that this approach has reduced the MAE and RMSE metrics by 19.86% and 5.26%, respectively, compared to the best-performing baseline model. Keywords: sentiment analysis; cross-attention; interactive graph mask attention network; multimodal.
Abstract: Sophisticated technology is required to evaluate college and university ideological and political education since it shapes student values, morality, and societal obligations. This paper suggests a cuckoo search-based extreme learning machine (ELM) model optimisation approach to handle this challenge. Using CS helps to maximise ELM model input weights and biases, hence enhancing stability and assessment accuracy. The work consists in three key investigations: In evaluation accuracy, CS-ELM performs better than other optimisation techniques and the benchmark ELM model. Second, ablation studies for every model component revealed the effects on the final assessment results of the CS optimisation algorithm, input weight optimisation, bias optimisation, and other factors. At last, time consumption comparison experiments reveal that in practice the CS-ELM model has low computational demand and great assessment accuracy. Keywords: cuckoo search; CS; extreme learning machine; ELM; ideological and political education; educational evaluation. |